The FURIFLOOD Project
Current and future risks of urban and rural flooding in West Africa – An integrated analysis and eco-system-based solutions
The German-African FURIFLOOD project, funded by the German Federal Ministry for Education and Research (BMBF), focuses on analyzing the impact of current and future flood events in both urban and rural regions of West Africa. By integrating scientific knowledge with case studies, the project aims to enhance understanding of future risks to human populations and the environment.
Within this project, a Decision Support System (DSS) has been established to create an online platform and dialogue forum for decision-makers in West Africa. This platform extends the existing LANDSURF platform to further investigate flood-related hazards and risks, particularly at the catchment scale but also on the continental scale.
The platform highlights the main outcomes of the FURIFLOOD project:
- Flood hazard severity maps for the Kumasi region and Ouémé river basin (see Chapter 2 for more details).
- Flood risk maps for the Kumasi region and Ouémé river basin, including information on exposure and vulnerability (see Chapter 3 for more details).
- Station-based return periods for daily precipitation extremes (2, 5, 10, 25, and 50-year) for current and future periods (see Chapter 4 for more details).
- Return value maps for daily precipitation extremes based on satellite data (Integrated Multi-satellite Retrievals (IMERG) for GPM), detailing 2, 5, 10, 25, and 50-year periods for both current and future conditions (see Chapter 5 for more details).
The Web Portal - DSS
What is a Decision Support System (DSS)?
- It's an interactive and flexible computer based system
- DSS's are used for the identification and solution of complex problems
- Provides information to support making the best possible decision
Note
Decision support is neither a decision proposal nor a decision itself. This portal aims to give solid and efficient information in a comprehensive way.
Spatial Decisions - SDSS
Our system can be described as a spatial decision support system (SDSS)
- Maps play a decisive role in decision support processes
- Usage of maps reduce decision time
- Increases understandability and accuracy of the results
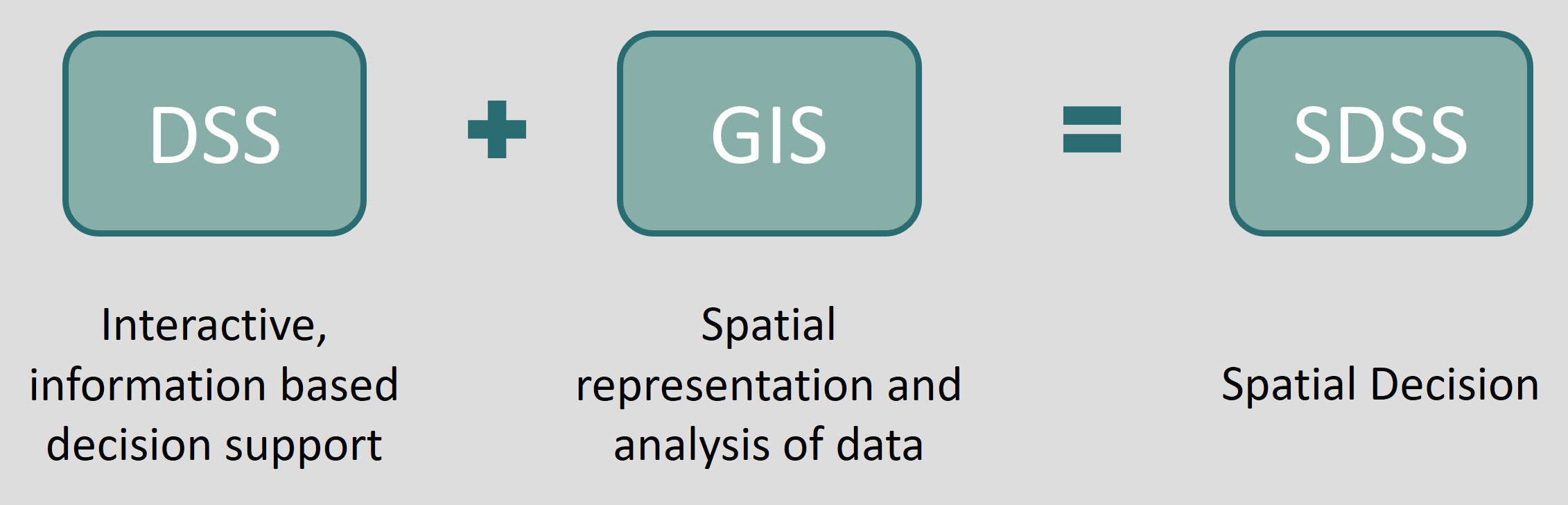
fig. 1: Scheme of a SDSS